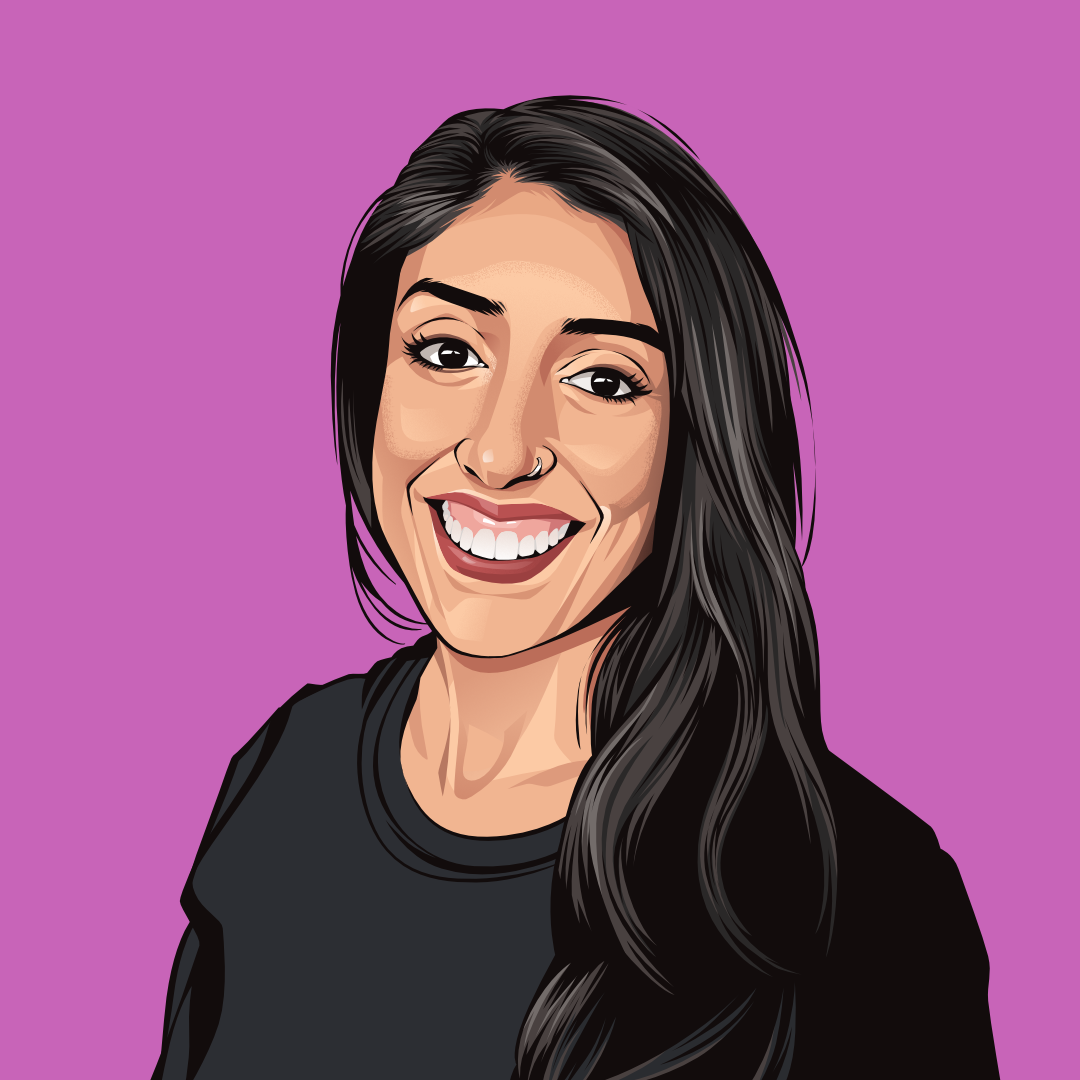
Unearthing insights from qualitative data is an arduous, manual process that typically requires human oversight. Teams sink hours or even days into analyzing and interpreting customer feedback across channels, rigorously combing through each piece of data to ensure they don’t miss anything.
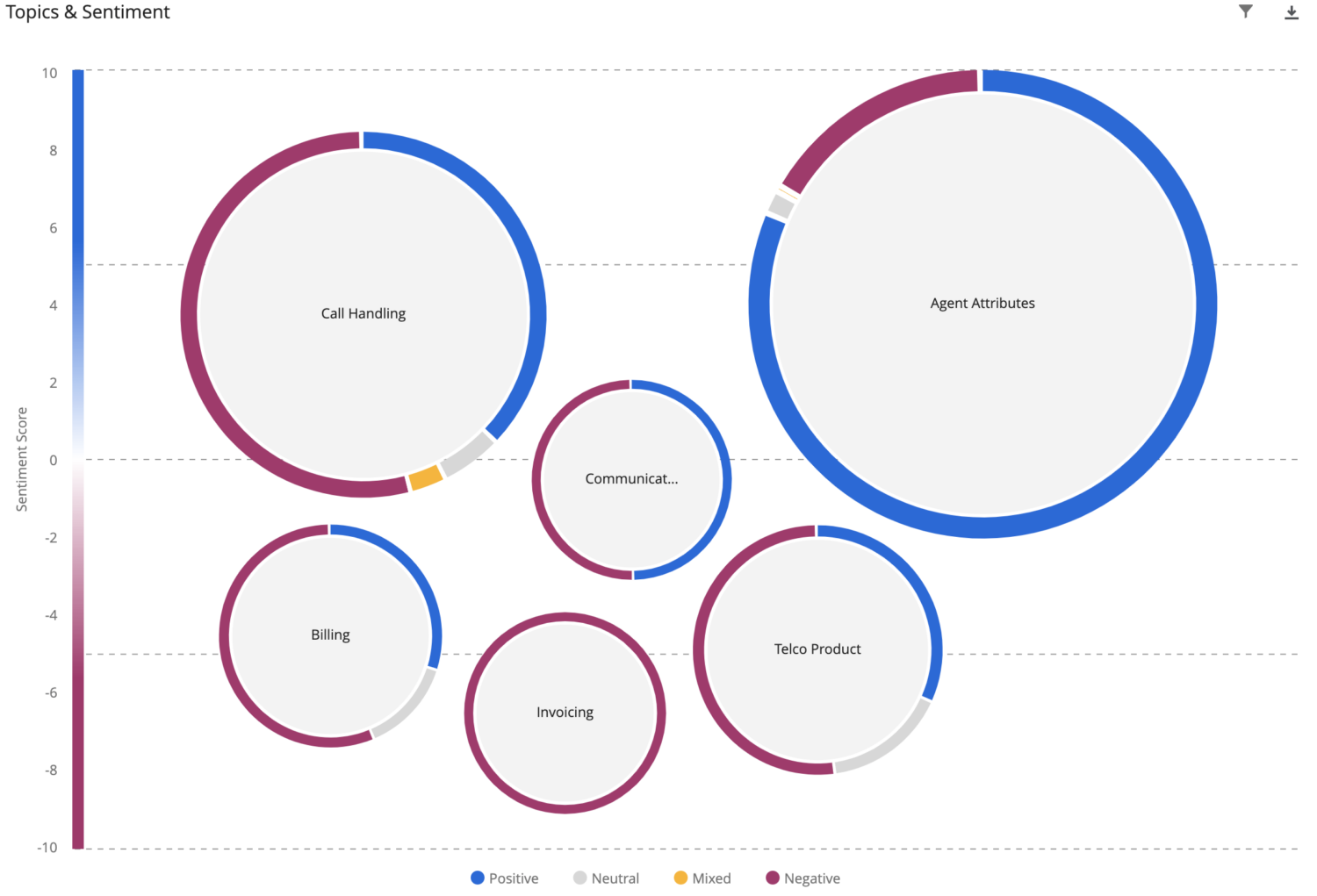
Today, there are many text-analytics tools in the marketplace that help with this process but they're generally unable to replicate true, human-level analysis. The majority of these tools simply quantify your qualitative data. What does that mean? These tools are able to tell you how many times your data mentions specific topics and layer in sentiment analysis to give scoring on these topics (positive, negative, neutral). So how does this fall short of real human analysis?
Consider this: if a large volume of reviews are talking about a feature limitation, an analytics tool might surface that there are a significant number of customers complaining about this particular feature and give it a high negative sentiment score with a big red flag. This would read as a problem with that feature that needs to be addressed immediately. However, a human analyzing the same data would see that it’s only your non-paying, free tier customers complaining about a feature limitation which was intentionally put in place to encourage users to upgrade. They would bucket this data into a non-urgent theme, in spite of its high volume, and instead focus on a churn risk for paying customers, even if that complaint volume is much lower.
So how can you streamline the analysis of your customer feedback to focus more time on actioning your insights? We’ll outline the seven steps of qualitative data analysis below and then show how you can automate this process using AI.

Boost customer satisfaction with precise insights
Surface the most urgent topics by telling our AI what matters to you.
THE PROCESS
Step one: Define the Questions
You likely start your process by defining what you were hoping to learn from customers, and what questions would get you that information. This sounds easy to the uninitiated, but this step can prove quite challenging – it can take hours to design the perfect questions. And if you’re collecting data from a large group of people, you’ll likely go with multiple choice questions because of the time and effort required to analyze broad, open-ended answers. This requires thinking through all the possible answers upfront and may even result in you missing the true reason behind some of your audience’s answers. But who has the time to analyze 500+ freeform answers?
What if there was a way to efficiently analyze large amounts of text, allowing you to do away with long-winded multiple choice surveys and instead ask a few open-ended questions? This is where AI can come in, significantly simplifying this step.
Step two: Collect the data
From there, you need to share your meticulously-crafted questions in whatever form is most applicable and collect the answers. If you’re lucky enough to have data from multiple sources such as product reviews, customer support tickets, surveys, etc., aggregating the data can be time intensive and costly. Your choices are: spend money on data aggregation software or spend dozens of hours using spreadsheets and pivot tables. Neither scenario is ideal.
Step three: Clean and sort the data
Next up – cleaning and sorting the data. As a part of this process, you’ll need to detect and remove all the noise. Humans are prone to making mistakes when collecting data, and data collection instruments may be unreliable, resulting in dataset errors. The errors are referred to as noise: everything from one-word answers that don’t provide value to answers that completely disregard the question asked. When dealing with thousands of pieces of feedback that inherently become repetitive, this is a high task ripe for human error.
Step four: Organize the data and cluster it into themes
After this is complete, the data needs to be organized and clustered into buckets based on the volume of response related to each topic. Most text-analytics tools stop here, but we all know this isn’t the final step in getting actionable insights from your qualitative data.
Step five: Analyze the data
This is likely the most time consuming step, requiring a lot of human oversight, and costly by nature as it’s most frequently completed by product managers. True human analysis runs deep and is incredibly difficult for a technology to replicate – which is why, until recently, no company has tried.
To show you what we mean, let’s dive into a feedback theme that the above-pictured text-analysis tool identified: billing. While the text-analysis tool tells you that the majority of the feedback is negative, it doesn’t unpack any of the other details surrounding this theme. Why do customers bring it up - are their bills inaccurate or are they simply having trouble processing their online payments? Is this an urgent issue that will lead to churn or a quality of life feature request? Where are they encountering their billing issues and who is primarily giving this feedback?
By leveraging the power of GPT-3’s natural language processing, you can dig into your data to get answers to these questions without requiring a ton of manual hours. An AI can provide a summary of the important themes directly from any data source (no more spreadsheets) with week-over-week changes, and go beyond sentiment scoring by analyzing how urgent the issue is based on the people delivering that feedback and the language used. Do your users discuss cancellation or use angry verbiage? Are the people giving that feedback your NPS Promoters? If so, the AI will take that all into account when delivering your weekly analysis and tagging it with the right urgency score.
Weekly reports pull in direct quotes from the feedback to validate the analysis, and leverage metadata to highlight traits related to that feedback (e.g. all users who reported billing issues were in Ohio and accessing our software on an iOS device). The AI combs through every piece of qualitative data to call out any blind spots (users who reported billing issues shared that the app had been repeatedly crashing), so that your team can know where exactly to focus. And all of this is written so articulately, you would never guess an AI was behind it.
Step six: Interpret the data
Now that the analysis has been completed, those PMs we mentioned before need to interpret the results, identifying issues and key actions the company can take to address customer feedback. As with analysis, a GPT-3-powered AI could take this step off your PM’s plate, and only leave them with the seventh and final step.
Step seven: Take action & measure
Finally, you and your team need to build an action plan, put it into effect, and then measure the results.
When done manually in its entirety, this work takes up significant time and resources, and even when a text-analysis tool is leveraged for steps 2-4, a large portion of the work remains.
Viable revolutionizes this process.
Viable’s GPT-3-powered AI handles steps 1 through 6 for you, delivering natural-language reports filled with analysis so actionable it’ll have you convinced a human wrote it. Customers of ours — like Latch — have leveraged our technology to free up their team’s time so they can focus on delivering a best-in-class experience, instead of being bogged down by manual analysis.
Pretty soon, we’ll be making step (7) easier by incorporating feedback loops into the software, enabling your team to seamlessly notify customers who reported a specific bug or issue that it has been resolved.
Let us help alleviate your team’s workload so you can focus on the important stuff – your product and customers. We’ll even process your first batch of data for free.
Start your free trial
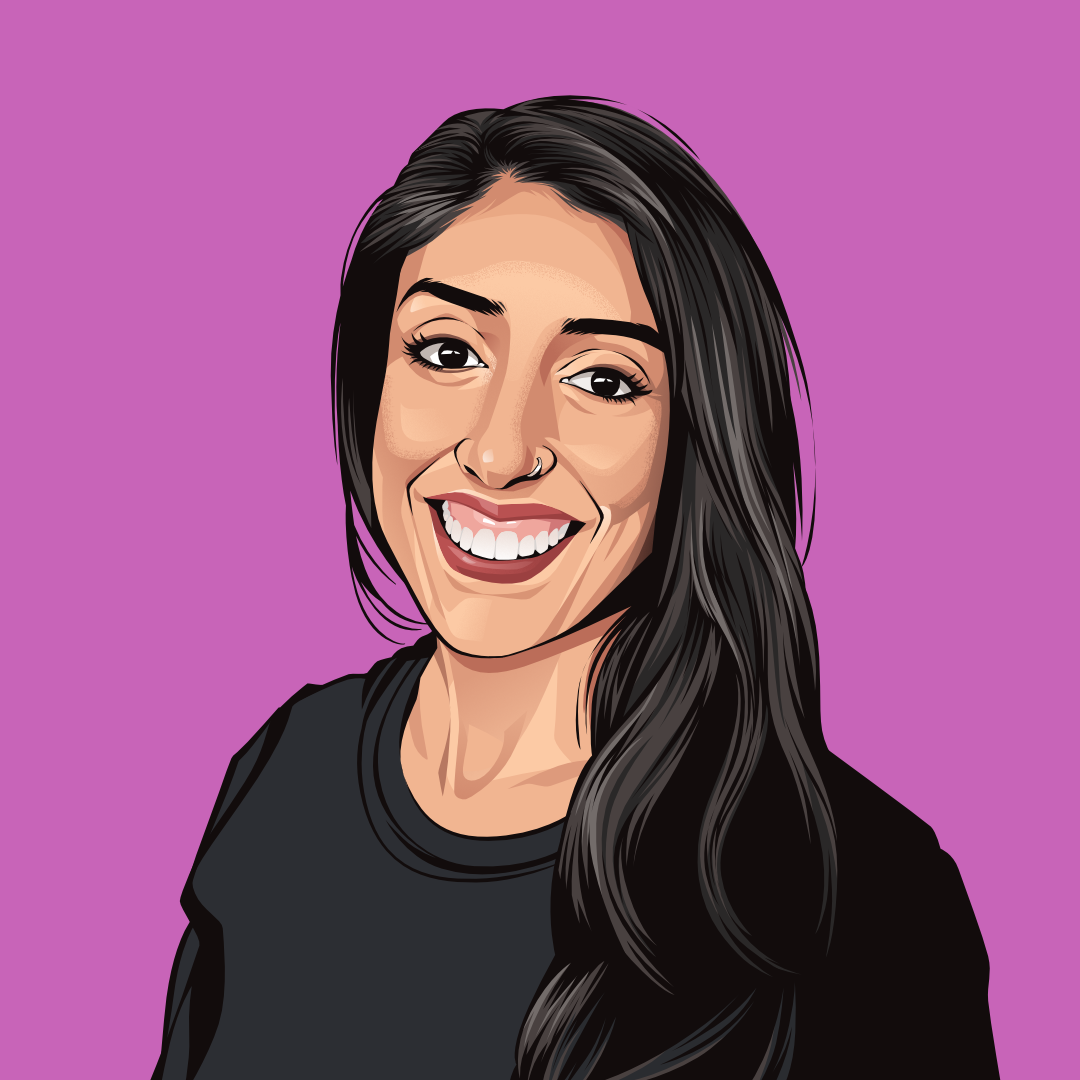
%20(10).png)
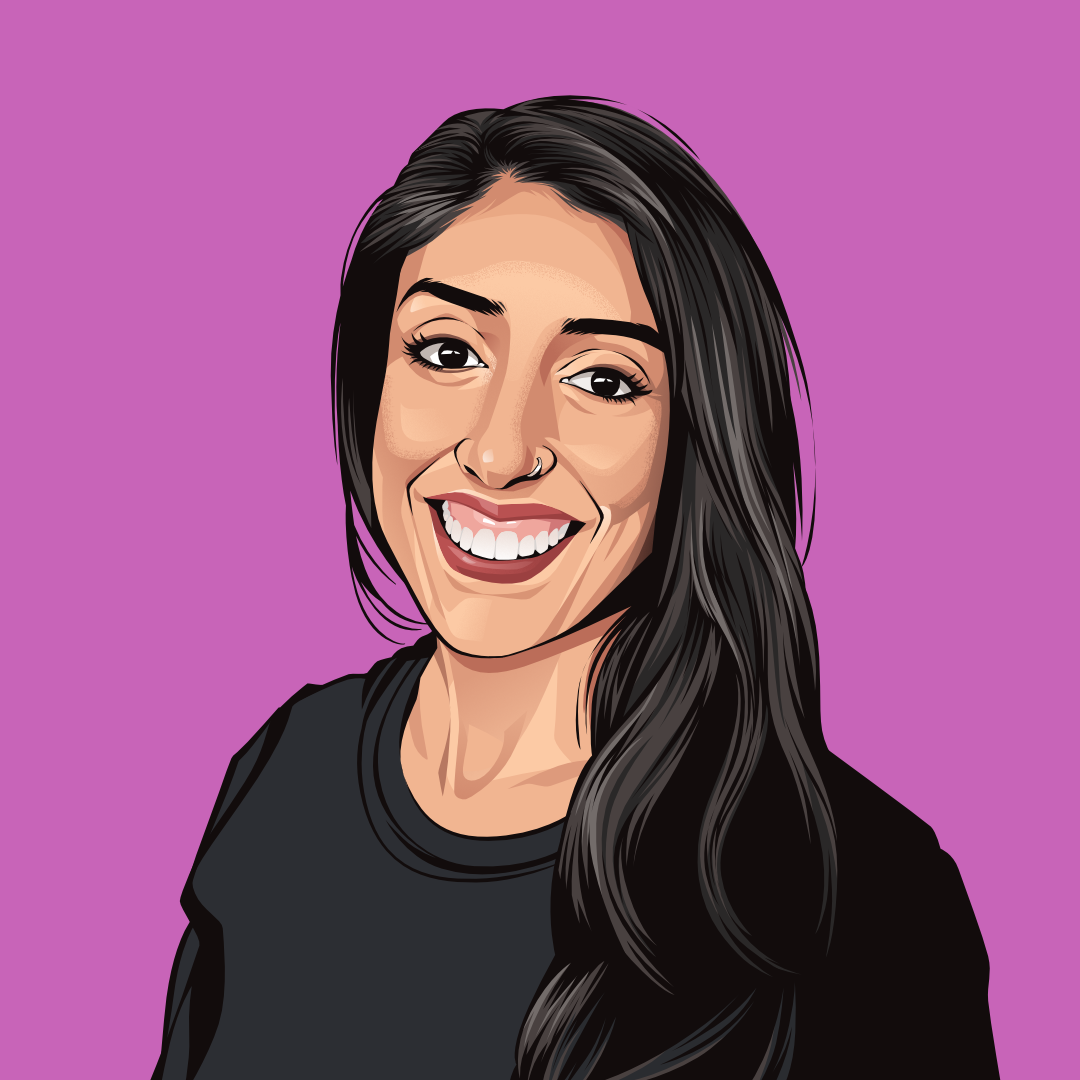
%20(9).png)
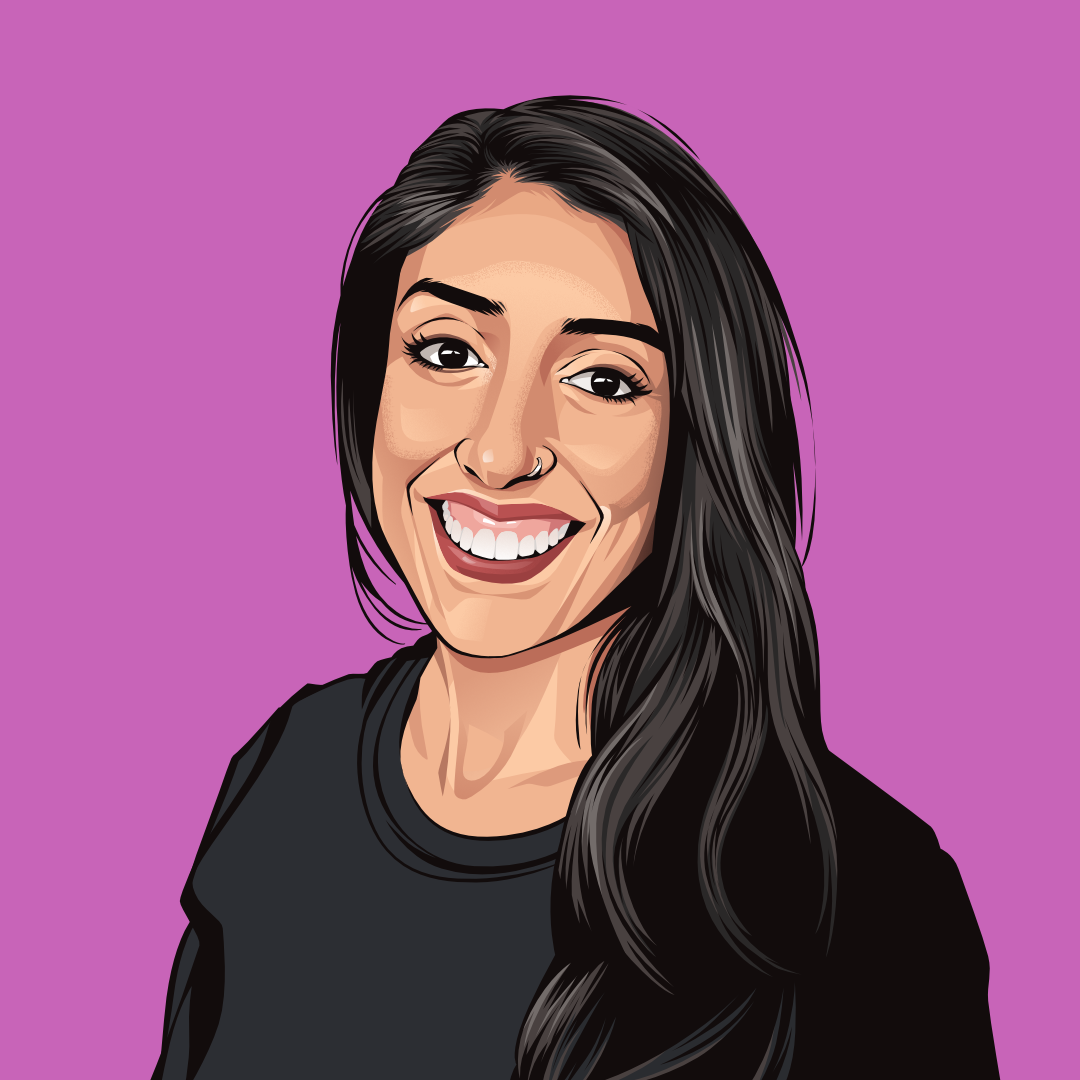
%20(8).png)
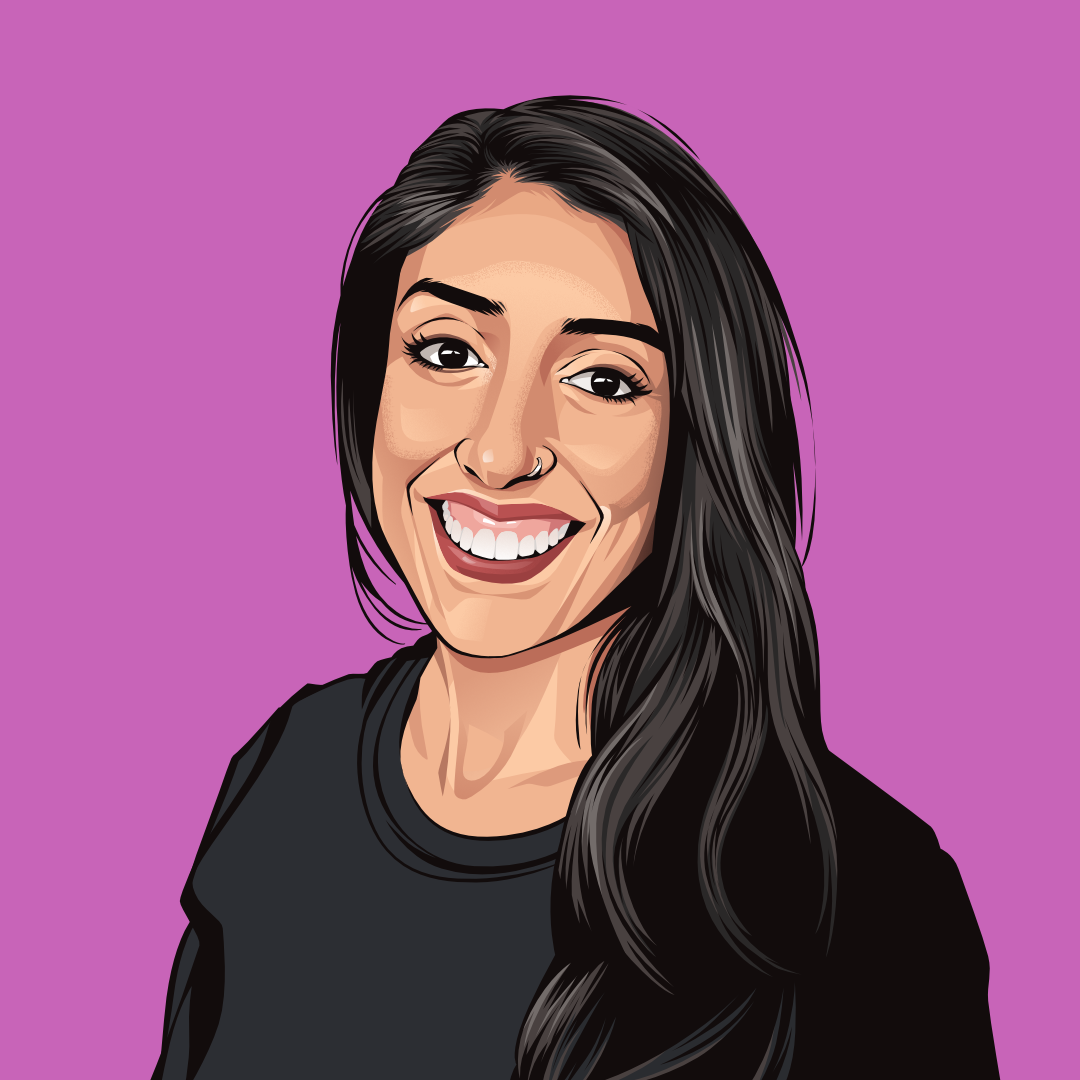