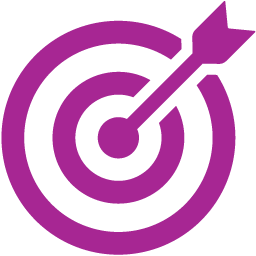
In part 1 of our guide on how to analyze customer surveys, we covered why customer surveys matter, what they look like, and how to set them up. In this second part, we cover the challenges of preparing customer survey data, how to prepare your data for analysis, and what to look for in specialized survey data analysis tools.
Let’s not beat around the bush: organizing qualitative data is hard work
The richest feedback from customers is open-ended text. From CSAT surveys (customer satisfaction), NPS surveys (net promoter score), in-app feedback requests, user research surveys, and more.
As much as you’d like to read every survey response, the reality is that you likely don’t have the time.
Let’s say you actually have time to read every customer survey response. Do you have time to tag each one by topic, do sentiment analysis, and segment them across customer traits in a neatly organized spreadsheet? Probably not. This is out of reach for most people. Especially when hundreds or thousands of survey responses are coming in weekly, monthly or quarterly.
If your product development cycle is short, congratulations! You’ve got a team of builders who can keep up with fast-moving ideas. Adding customer feedback analysis to that cycle shouldn’t slow it down. It often does because text analysis is a manual and time-consuming task.
But there are tools to structure survey responses for analysis, you say. Yes there are. With a little preparation, you’ll get a lot of use out of these tools.
How to prepare your data for qualitative data analysis
Before finalizing your survey design, capture the data you’ll need for analysis. Include as much useful information upfront so you can slice and dice later. This includes segmentation and survey-specific data we call metadata (data about the data).
Segmenting customers is common in qualitative data analysis. Many customer traits are pre-known per customer, such as customer type, location, job title, company type, or type of device used.
Other customer traits aren’t known upfront but can be captured via survey questions like a customer’s intended or past product usage, repurchase interest, or use case (e.g. the problem they’re trying to solve).
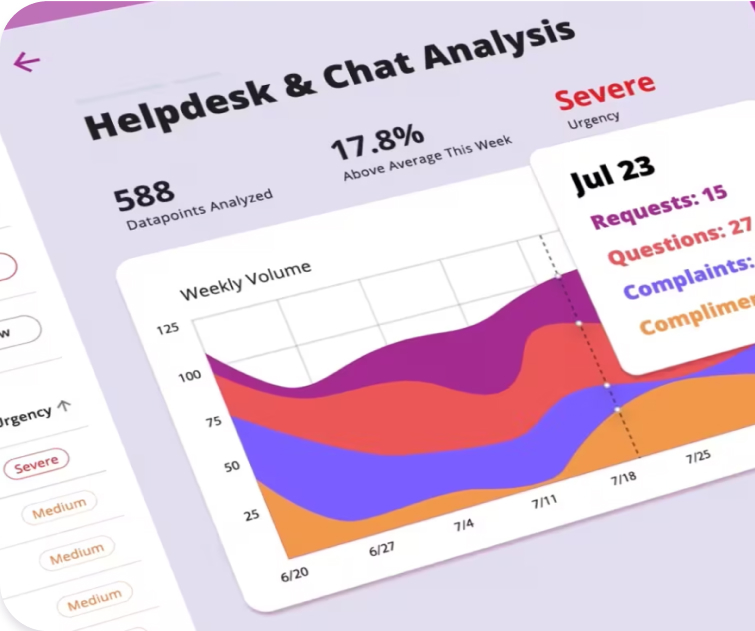
Boost customer satisfaction with precise insights
Surface the most urgent topics by telling our AI what matters to you.
Customer traits for segmented analysis
The types of customer data you can include in your analysis are:
- Customer traits – demographics, job title, geography, behavior, usage intent (e.g. personal vs. business), device use (iOS vs. Android), etc
- Product attributes – such as product line, product category, subscription tiers, service packages
- Survey metrics – NPS or CSAT scores
Survey metadata for more precise filtering
Additional data that will make analysis more precise often contains:
- Timestamp – to analyze feedback over time
- Custom fields – custom tags in the source of the data
- Prompts – the questions asked in the survey
You don’t need fancy apps to gather all this information. Putting it in a CSV file is not only sufficient but common.
What to do when it’s time to analyze surveys
Once you’ve prepared your data, it’s time to analyze it. There are a few ways to do this:
- Do all aspects of data analysis yourself, manually
- Build your own in-house analysis engine
- Use tools that specialize in survey data analysis
We don’t recommend the first option for reasons we’ve stated before. And, unless you have very unique needs along with a significant budget and dedicated resources, the second option is not feasible for most companies.
Option 3—using tools that are built specifically for survey data analysis—will save you time, money, and frustration.
Choosing a qualitative analytics tool to analyze your survey data
A crop of new specialist tools for qualitative data analysis, including Viable, are taking advantage of impressive new language models to make text analysis easier than ever. They’ll cut hours otherwise spent on extracting insights from open-ended answers to follow up questions in NPS, CSAT, and other types of surveys.
Some things to look for in these tools for analyzing customer survey data:
- Easily syncs with your customer survey tools for ongoing, hassle-free data import
- Requires little if any additional data preparation
- Has flexible dimensions such as date range, product/customer traits, and data sources
- Can be used by anyone, regardless of technical skill set
The last point is important to us. At Viable we passionately believe that getting insights from a tool shouldn’t require jumping through hoops to translate your question into...a more technical query.
A good qualitative data analysis tool should enable you to ask a question as soon as you think of it and get an answer immediately, in plain language. If you have to interrupt your train of thought to force your question into a specific set of technical parameters, then the tool is going to slow you down.
Lastly, we encourage you to think about how you like to conduct analysis of your customer surveys. Chances are you’ll want to:
- Measure customer satisfaction or sentiment across time
- Identify the top complaints, questions, compliments, and feature requests from customers on a regular cadence (e.g. weekly)
- Ask specific questions about any issue to dig deeper, quickly and easily
- Share findings with your colleagues without a lot of extra work
A tool that uses an advanced language model will go a long way to ensuring accuracy and quality in your analysis. A tool that doesn’t require you to translate your questions into specialized queries will make your data accessible to everyone in your organization that cares what customers think.
Key takeaways: a bit of data prep goes a long way
Analyzing customer surveys is easier than it’s ever been thanks to tools that specialize in qualitative data analysis. This is good news for companies looking to shorten feedback loops, iterate quickly, and stay competitive. Below is a summary of how to get the most out of customer surveys from our 2-part guide.
Have a mix of closed and open-ended questions. Make it easy but also give customers a chance to express themselves.
Limit the number of questions in your survey to increase chances of completion. No more than 5, ideally maximum of 3 questions for NPS surveys.
Know what information to gather for your survey analysis: timestamp, traits, metadata if needed, and individual identifiers.
The richest feedback comes from answers to open-ended questions. Find a tool that uses advanced language models that can parse open-ended text at scale so you don’t have to.
Advanced language models aren’t enough. To make customer survey analysis—or any qualitative data analysis—accessible for all users regardless of technical skill, look for a tool that directly supports natural language questions. Your tools should not slow you down by making you translate questions into technical queries.
Got survey responses you'd like to analyze? Talk with us.
If you want to analyze customer survey responses you’ve been collecting, we'd like to help.
We believe you should spend no more than a few minutes preparing your data, freeing you up to find insights. When you have a question, you should get answers instantly. No query translations. It should be as simple as asking a question.
If you’d like to learn more or see it yourself, let's connect.
Talk with us.
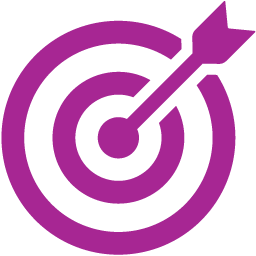
%20(10).png)
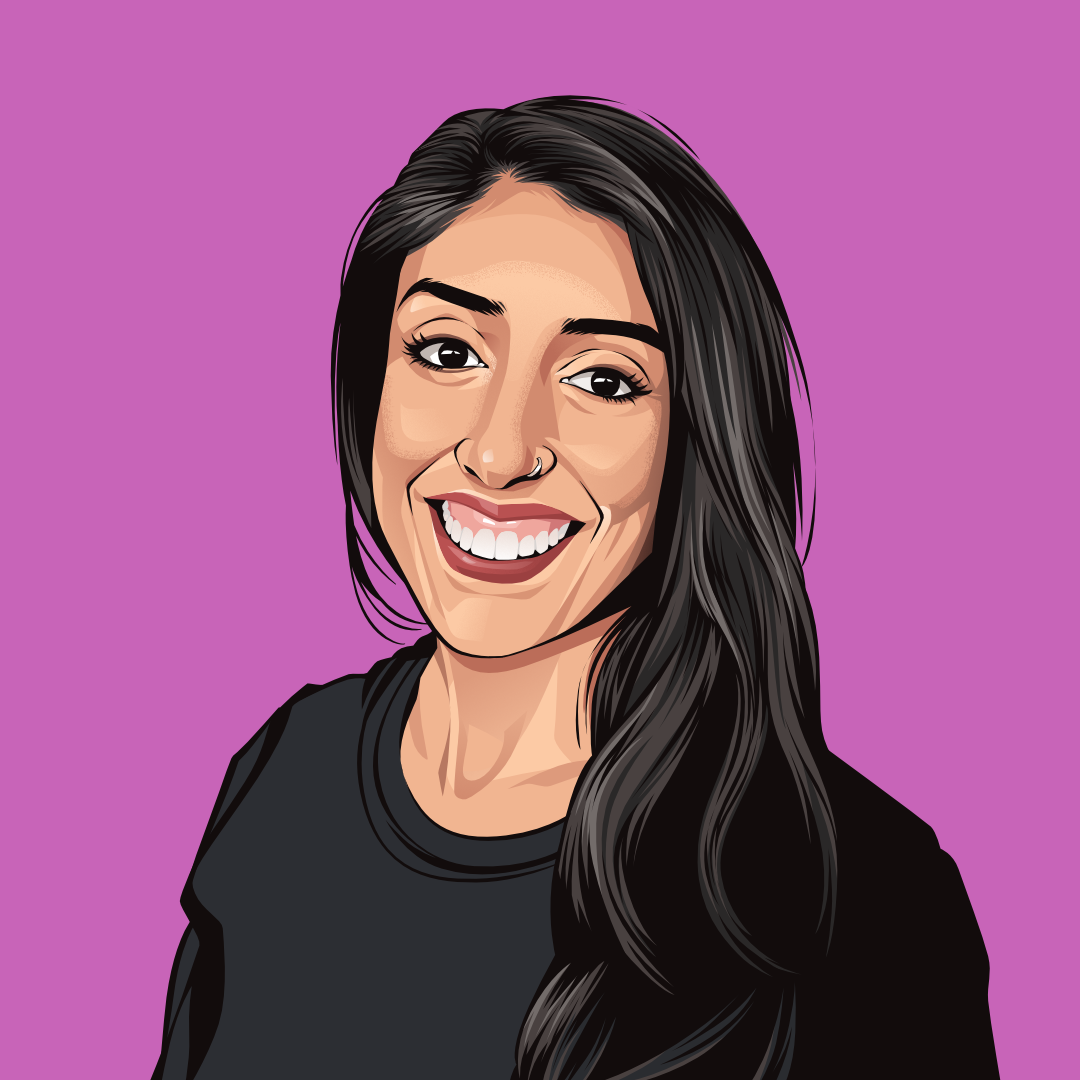
%20(9).png)
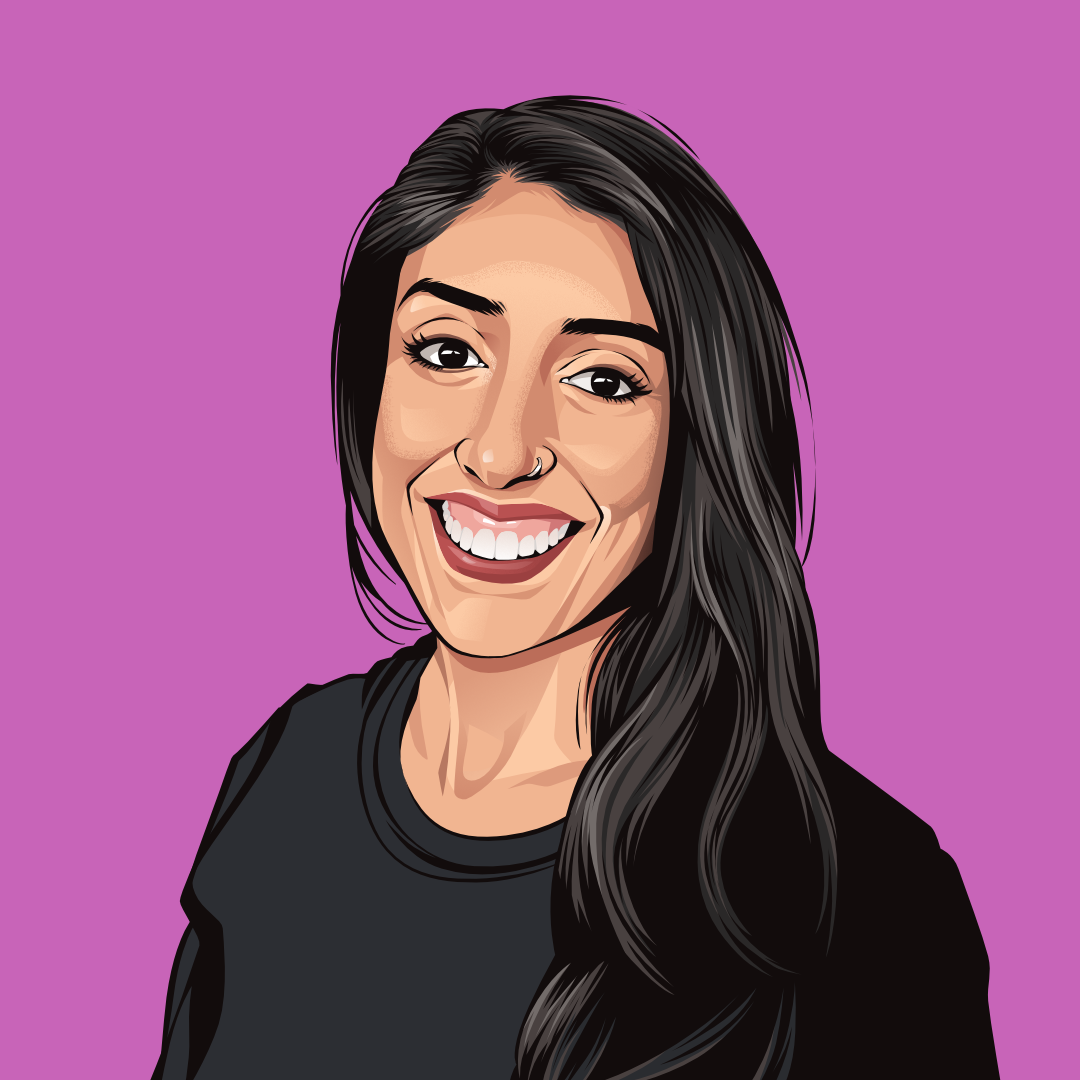
%20(8).png)
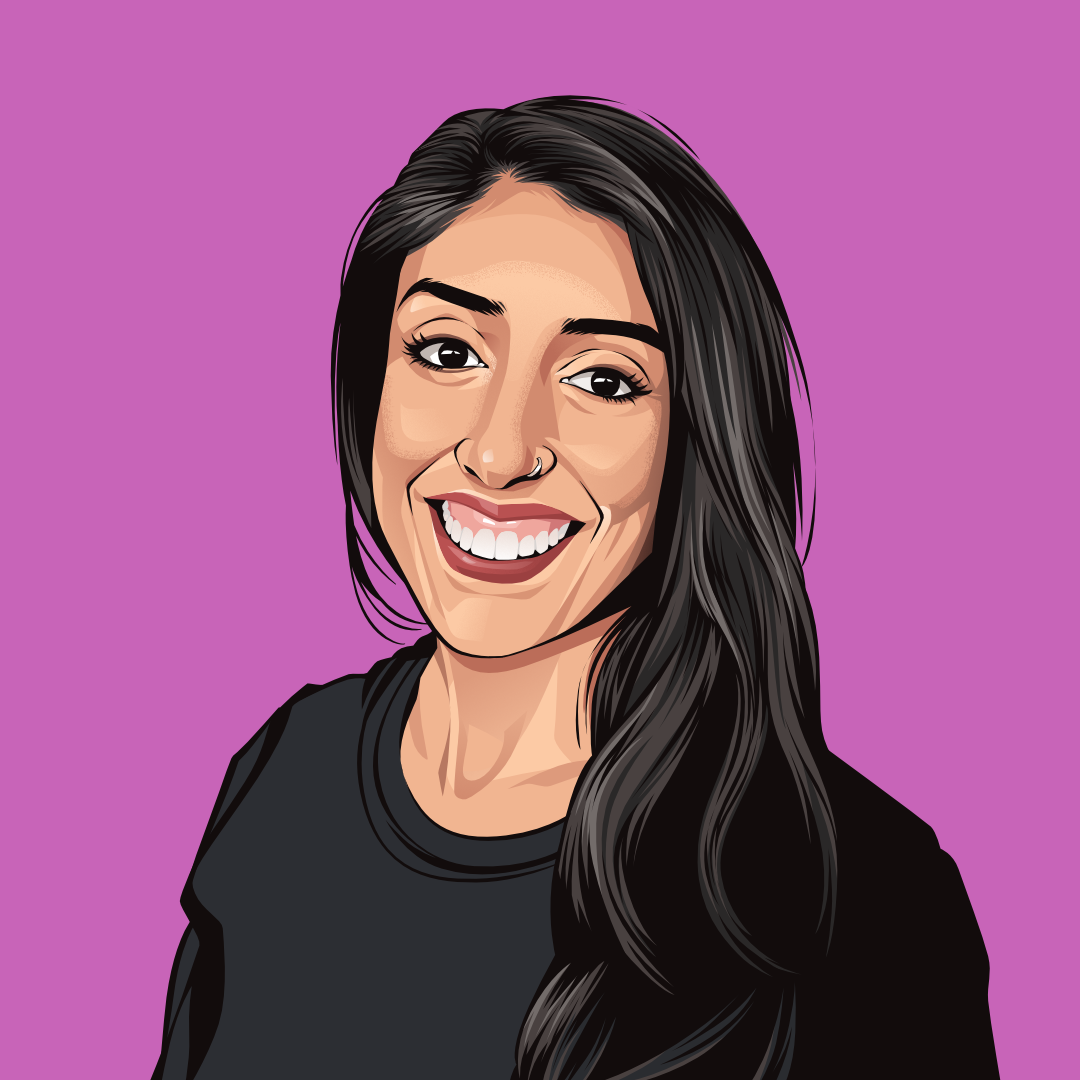