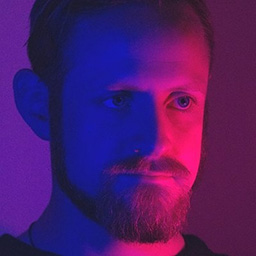
You've got a lot of customer feedback living in your support tickets from apps like Zendesk and Intercom. Someone at your company spends hours making sense of that data and reporting those insights to management, product, marketing, sales, and operations teams.
We spent the last year at Viable talking to heads of customer support and we learned a lot. It takes a lot of manual effort to tag, sort, and mine insights out of all that data.
The biggest challenge is dealing with the volume of feedback. But it's also qualitative in nature, which makes it difficult to structure. Teams have to read through feedback one by one, identify themes, and tag them. Someone may even have to (manually) label the data by sentiment or emotion.
The manual effort involved makes it difficult for most companies to get useful insights from customer feedback quickly.
We launched Viable to solve this.
Viable structures customer feedback so you can get answers fast. We automated the sorting and tagging process using the most advanced language model that exists today, GPT-3. This AI model was released in 2020 by artificial intelligence research lab OpenAI.
The GPT-3 model is exceptionally good at writing language like a human. With a handful of data points and minimal instruction, the model interprets text with impressive accuracy in a matter of seconds.
It’s perfect for the problem we were looking to solve.
We applied GPT-3 to do two things well:
- Sort through customer feedback and identify themes the way a human would
- Pull insights from aggregated feedback and summarize it in human language
Our criteria was that it had to be automatic and fast. Below is how it works.
Automated sorting and tagging that's highly accurate
Viable assigns tags, emotions, and sentiments to each piece of customer feedback, or data points, such as:
“I need to edit my order because the checkout flow didn't let me edit my address. Can you help me with that?”
Or
“Checkout takes way too long to load. This isn’t usually a problem, but when you have items that sell out fast it can be annoying when the item in your cart sells out before checkout loads.”
Viable would assign the following to these data points:
Tag: checkout
Emotion: frustrated
Sentiment: negative
It happens near instantly because GPT-3 is especially good at correctly identifying themes from human language. That’s the first way we applied GPT-3.
Useful summaries that knocked our socks off
With all customer feedback structured and ready for analysis, the next part is probably the trickiest: getting insights out of it.
What’s the most common way to get insights? We think it’s by asking questions. Natural-sounding ones, the way you would ask a colleague.
Viable takes your question, let’s say, “what’s frustrating for our customers about the checkout experience?”, and surfaces only the customer feedback that matches.
At this point, GPT-3 does the second thing we wanted it to do well: write a human-sounding summary out of all the matching data.
It would look this:
“Customers are frustrated with the checkout flow because it takes too long to load. They also want a way to edit their address in checkout, and they'd like to be able to save multiple payment methods.”
(This is a real answer generated from the examples in the previous section.)
It reads like a human wrote it, which makes it easy to share across teams.
In fact, even after weeks of using GPT-3, we’re still impressed with how well it writes!
A simple yet powerful product
When looking for insights, you should be able to ask as many questions as you want and analyze data in multiple ways. With filters, date ranges, customer segments, and sentiment/emotion analysis, Viable makes it easy to search for answers.
A simple setup is also table stakes. The thought of integrating a new tool makes everyone cringe. That's why we made app integrations in Viable easy, requiring just two clicks.
Underlying all that simplicity is an AI language model 10x better than any other. As product builders, we know the struggle of getting insights from qualitative data. GPT-3 changes the game in a major way. We find that asking questions and getting accurate, human-like answers in seconds is pretty magical. We think you will too.
Who we are
My brother and I have built product, design, and engineering teams at well-known, fast-growing companies for the past decade. We’ve been building solutions for clients for even longer than that.
We like hard problems. Organizing qualitative customer data was a particularly hard problem, and we’ve dealt with this problem at every company we’ve been a part of.
We think the honest feedback that customers are willing to share via support channels is an underused source of product and brand insights. We built Viable to tap into this uniquely well.
That said, we want to hear from you. If Viable seems useful, or if there are use cases we haven’t thought of, let us know.
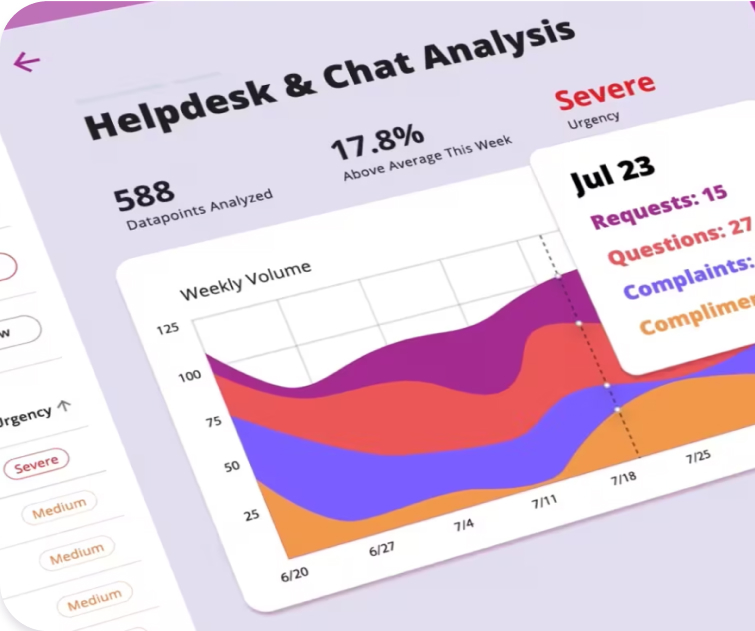
Boost customer satisfaction with precise insights
Surface the most urgent topics by telling our AI what matters to you.
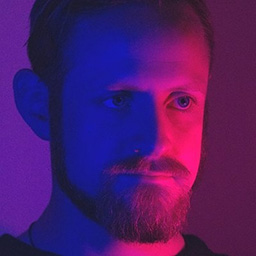
%20(10).png)
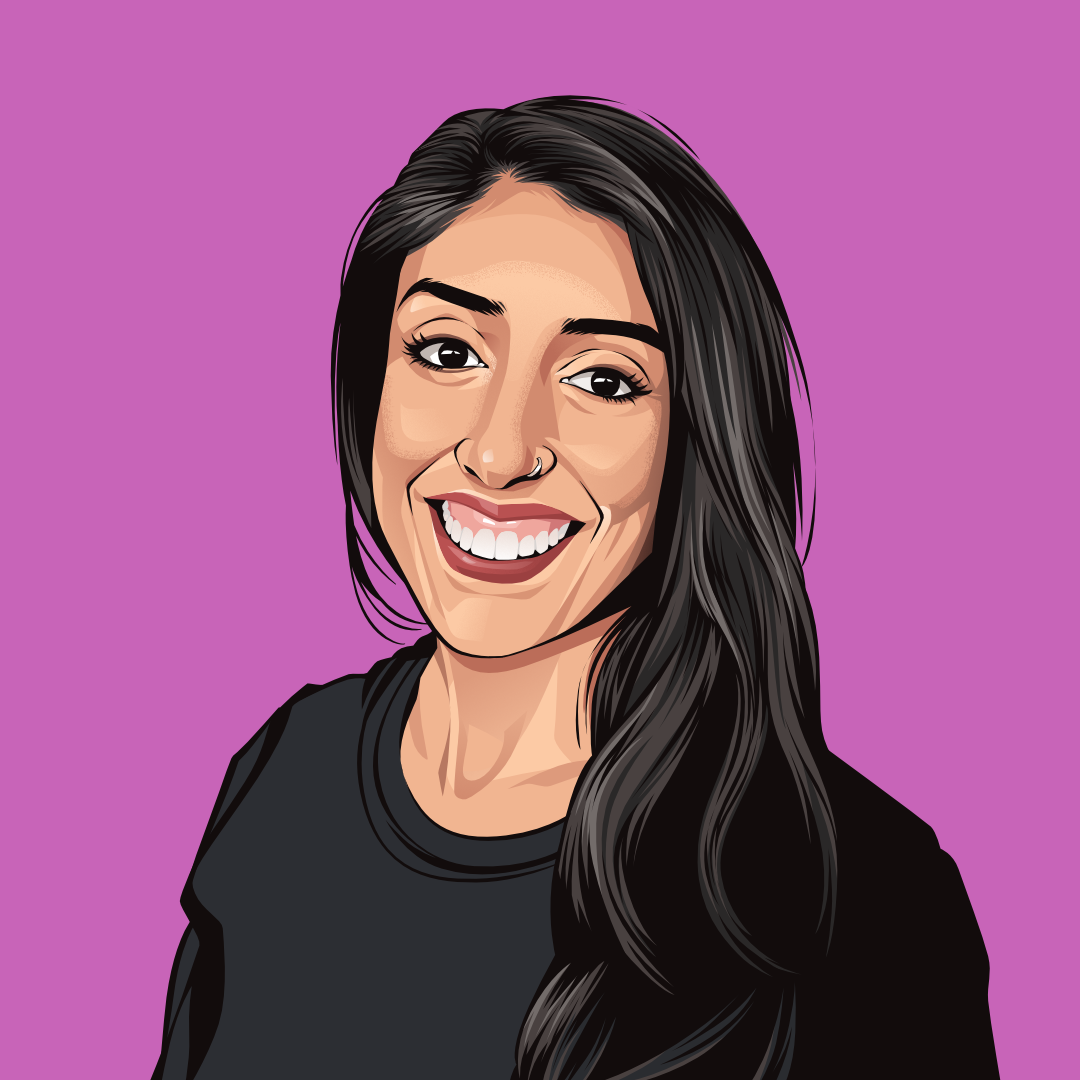
%20(9).png)
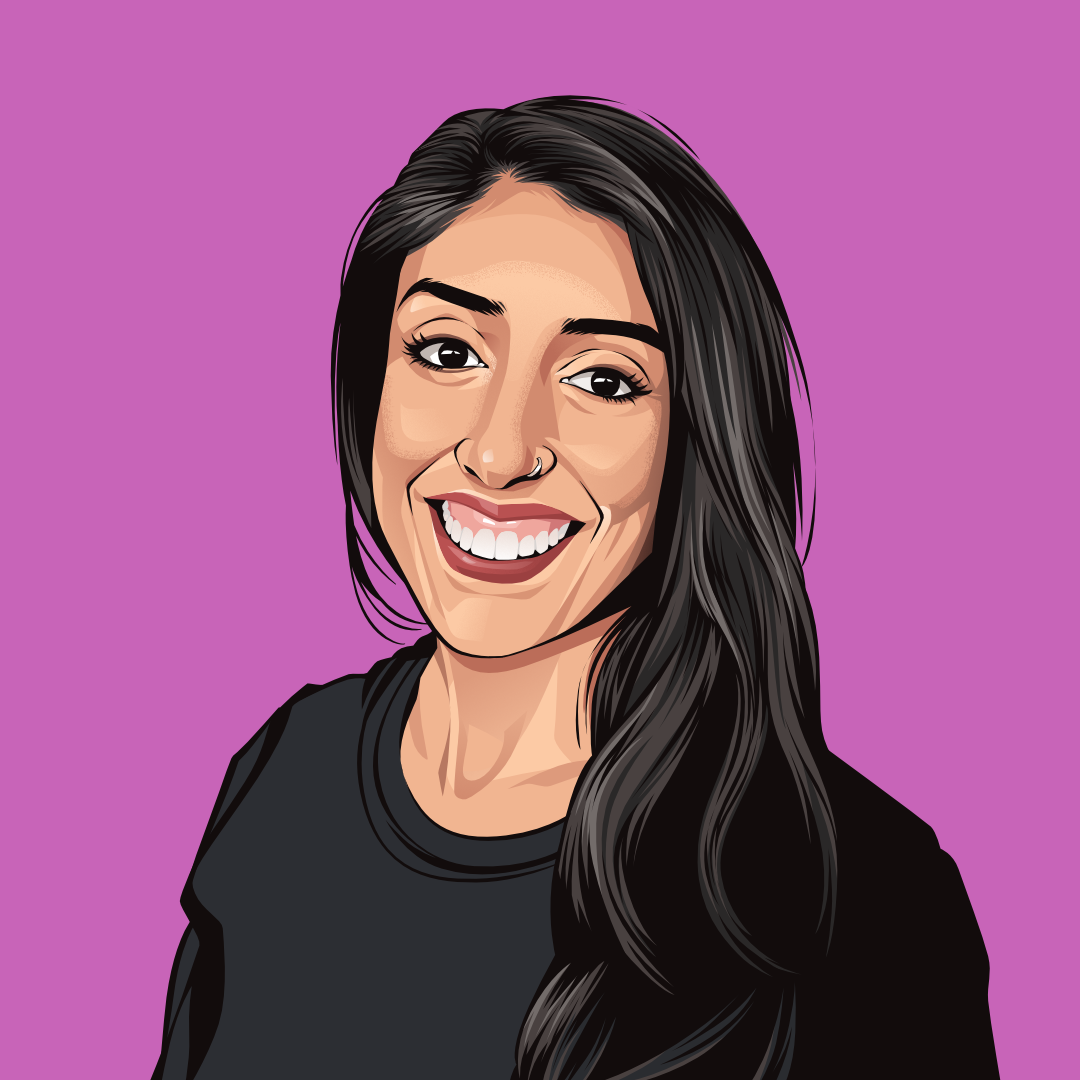
%20(8).png)
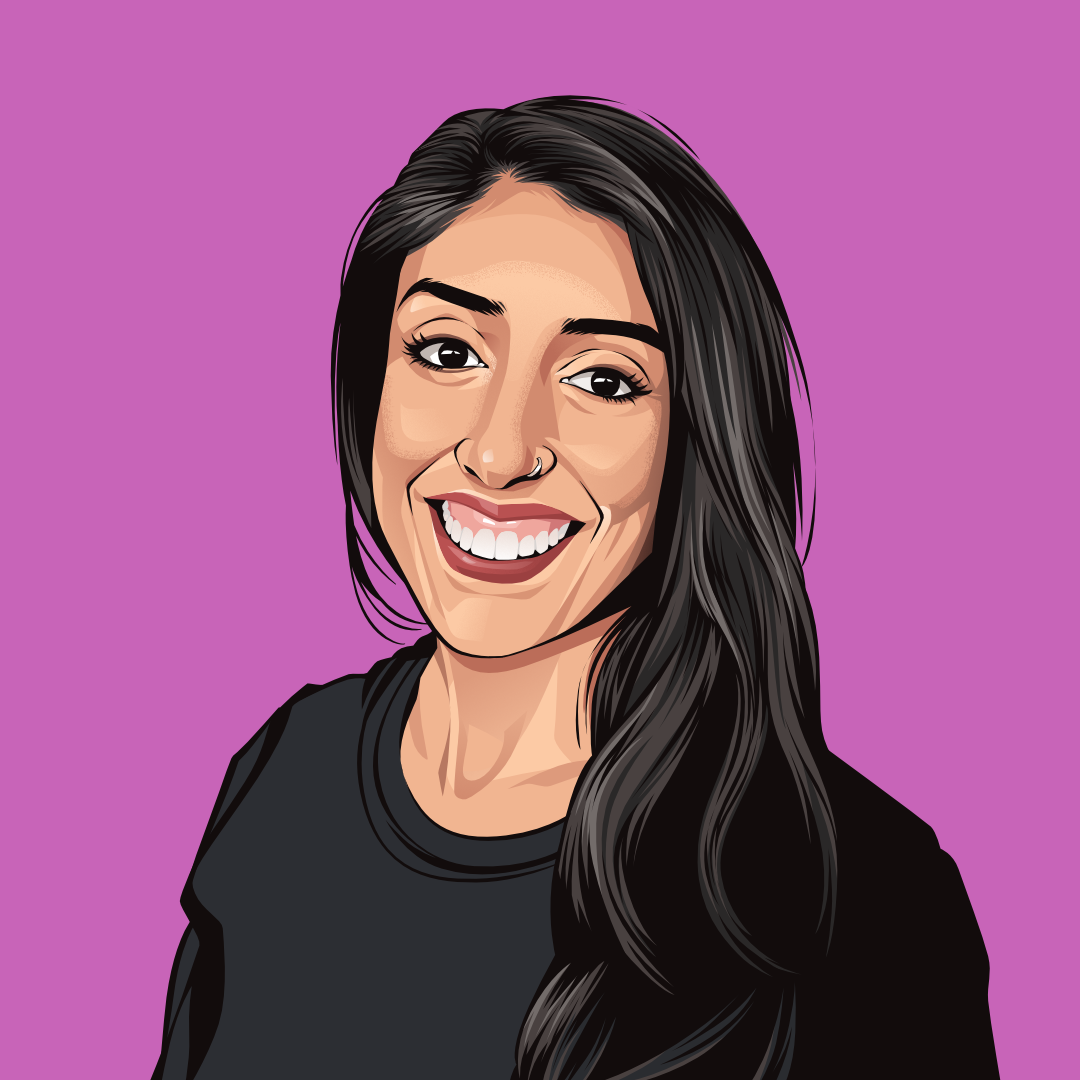