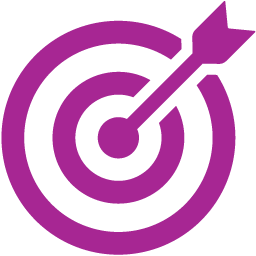
What is text analysis
Text analysis is the process of identifying meaning from text in a methodical way. It’s often done with sophisticated algorithms and machine learning.
Anyone in a business role has probably analyzed text using their brain and a spreadsheet to classify themes or topics, sentiment, and even emotion from text. This type of manual text analysis is common in companies large and small looking to the voice of the customer for insights.
Companies use text analysis to find insights in their customers’ qualitative feedback from surveys, user experience research, helpdesk tickets, app store reviews, support requests, or social media chatter. Unstructured text in qualitative feedback can be organized and analyzed using methodical techniques to find the insights you’ll use to improve your products and customer service.
Consider this example of customer feedback for a clothing company:
I recently purchased a pair of shorts from your company and I’m glad to report they are comfortable. The material is very nice and the waistband fits just right.
Text analysis would identify the following keyphrases from this example:
Shorts
Comfortable
Waistband
Going beyond keyphrases: under an area of text analysis called topics or topic clustering, the entire entry would be categorized as being about fit.
What is sentiment analysis
Sentiment analysis is an application of text analysis that measures the feeling behind a comment. That feeling could be positive, negative, or neutral.
Sticking with the customer feedback use case, sentiment analysis gives you more context around a piece of feedback beyond the keyphrases or topics. For instance, in the example above:
I recently purchased a pair of shorts from your company and I’m glad to report they are comfortable. The material is very nice and the waistband fits just right.
Sentiment: Positive
Sentiment analysis helps you gauge trends across a large volume of feedback. If there’s a spike in negative sentiment, your customer service team would want to dig deeper to figure out what the problem is.
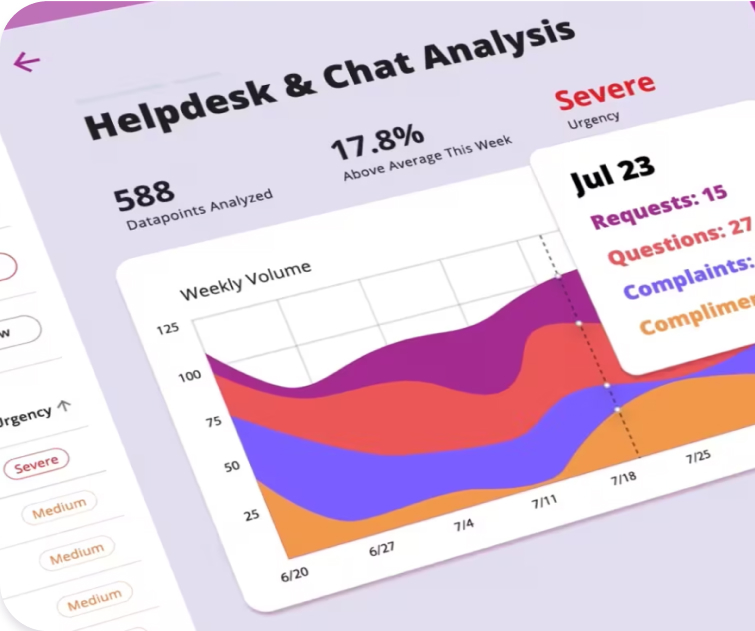
Boost customer satisfaction with precise insights
Surface the most urgent topics by telling our AI what matters to you.
Why text analysis matters for the customer experience
Customers will let you know their feelings, thoughts, and suggestions for improvements via product feedback forms, customer surveys, social media, or online reviews. Product teams often pour through these comments looking for ways to improve their offerings.
For customer-centric product teams, qualitative analysis is such an important part of the feedback loop that it can’t be skipped. Slack’s CEO and co-founder, Stewart Butterfield, has talked about the central role qualitative feedback plays at Slack:
“We’re pretty fastidious about tagging all of these incoming messages, collating and entering and retaining the data that people are sending us.”
Tagging and collating feedback are components of text analysis and overall qualitative analysis. These tasks are often manual, especially in the early days of a business. As companies become more established, volumes of qualitative feedback become too large for manual text analysis; dedicated tools for automating text analysis become useful.
Qualitative analysis tells you the why behind the what
Both quantitative and qualitative analysis of customer feedback can help you diagnose and address problems in your business.
Quantitative data tells you what customers do. Quantitative metrics that tell you what customers do include:
- Churn
- Conversion
- Usage or engagement
Engagement is a common metric for tracking the health of your business. It can be measured in a number of ways depending on your business model:
- Monthly active users
- Reports generated
- Messages sent
- Queries conducted
- Tasks completed
Engagement metrics can vary depending on your business. The important thing is tracking how valuable your product is to your customers in their workflows. (See more examples of quantitative vs. qualitative metrics for user experience research).
Once you know what customers are doing, you’ll want to know why they’re doing it so you can make decisions that decrease, maintain, or increase those behaviors. Qualitative feedback is where you’ll find the why behind the what. It is where customers share their frustrations, elations, or disappointments about their experience with your product or service.
Text analysis makes it possible to get insights from unstructured written feedback. That process is faster and easier when it’s automated. With ongoing text analysis at scale you can learn:
- What the most mentioned topics or themes are
- The mood or sentiment in feedback cumulatively or individually
- Your customers’ most frequent complaints or issues
- What customers most like about your product
- The most frequently asked questions
- The most frequently requested features
- Areas of friction in the onboarding process
- How well the product matches user expectations
- Whether a feature is working as intended
- Unexpected usage patterns
- Bugs that need to be fixed
How text analysis helps feedback loops
Product development cycles are getting faster. Many companies are finding themselves enabled to iterate and release new products more frequently. Customer feedback loops need to keep up with faster release cycles to ensure product decisions account for the voice of the customer.
Similarly for voice of the customer programs, studies show that companies who value the customer experience grow 5.7 times faster than laggards. Using insights from customer feedback can help improve customer satisfaction scores, retention, conversion, upsell, and other indicators of growth.
With text analysis built into feedback loops to inform product development and voice of the customer programs, you can:
- Test new or updated features
- Keep a pulse on how customers navigate your product
- Understand why customer behaviors change
- Pinpoint the source of customer excitement, frustration, disappointment, or indifference
- Identify and respond quickly to anomalies not captured by quantitative metrics
- Spot underlying causes of friction and drive faster issue resolution
What to look for in customer insights software that use text analysis
There are many technical guides that deep dive into the technical definitions of text analysis from a natural language processing and machine learning perspective. Our own machine learning experts at Viable are well versed in the pros and cons of different text analysis techniques.
For business users, we recommend considering the following when assessing text analysis capabilities in customer insights software.
Understand what is and is not included in the solution. You want to understand what the software's capabilities and features can help you achieve. Ask questions such as:
Is the solution designed to help your full team or just a few users get access to insights?
How easily can you get to the actual user comments from a question or search?
Can you ask as many questions as you want until you get the answer you need?
Does the vendor clean up noisy data for you upon data import?
Are any automated reports included?
Are trended views or dashboards included?
What kind of support do you get per price package?
How many data points can you process with each plan?
If you have one-off datasets such as surveys, or user research interview notes you’d like analyzed, ask if that’s a capability they can handle.
Dig into the system being used. Find out how topic modeling, sentiment analysis, and emotion analysis work. Ask for accuracy metrics, comparisons based on customer proof points, and anything else that will help you understand the underlying technology.
Beyond looking at the technology being used in the system, assess whether the ultimate output goes beyond tagging text. An advanced solution should also identify the underlying problems, possible causes and solutions, and the magnitude of severity. In other words, a complete analysis of feedback.
Assess whether the tool helps you add more value to your organization. Do the features help free up your time so you can get insights faster? If so, by now much? Think through how many tasks you undertake when analyzing qualitative feedback today and how many of those tasks are replaced by the software. Additionally:
Does the product help identify real answers from the qualitative feedback?
Does the product categorize the data in ways that help provide answers to your teams?
If you’re looking for a report that you can easily forward to your colleagues, find out what it takes to build such a report in the tool.
If asking iterative questions to find new insights is valuable to you, find out if the tool supports this use case.
Try it out with a few different datasets. If the vendor offers a free trial, give it a whirl with different data sets. This gives you a chance to see the quality of the text analysis, how easily you can connect multiple data sources, and how the vendor handles data quality and noise reduction per source.
Identify whether the tool is designed for business teams. If you’re looking for a text analysis and insights tool that’s easy for anyone on your team to use, make sure to thoroughly assess the user interface, reporting capabilities, and quality and completeness of the insights generated. Ask yourself if the output you get from a few keystrokes is something you can share with your colleagues as is.
Viable helps you easily get insights from qualitative feedback
Looking for qualitative feedback analysis with powerful text analysis capabilities that save you time? Need a solution that lets you ask natural language questions and doesn’t require that you create technical queries? Viable might be the solution for you.
Connect with us
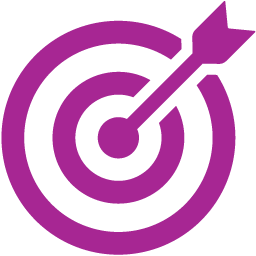
%20(10).png)
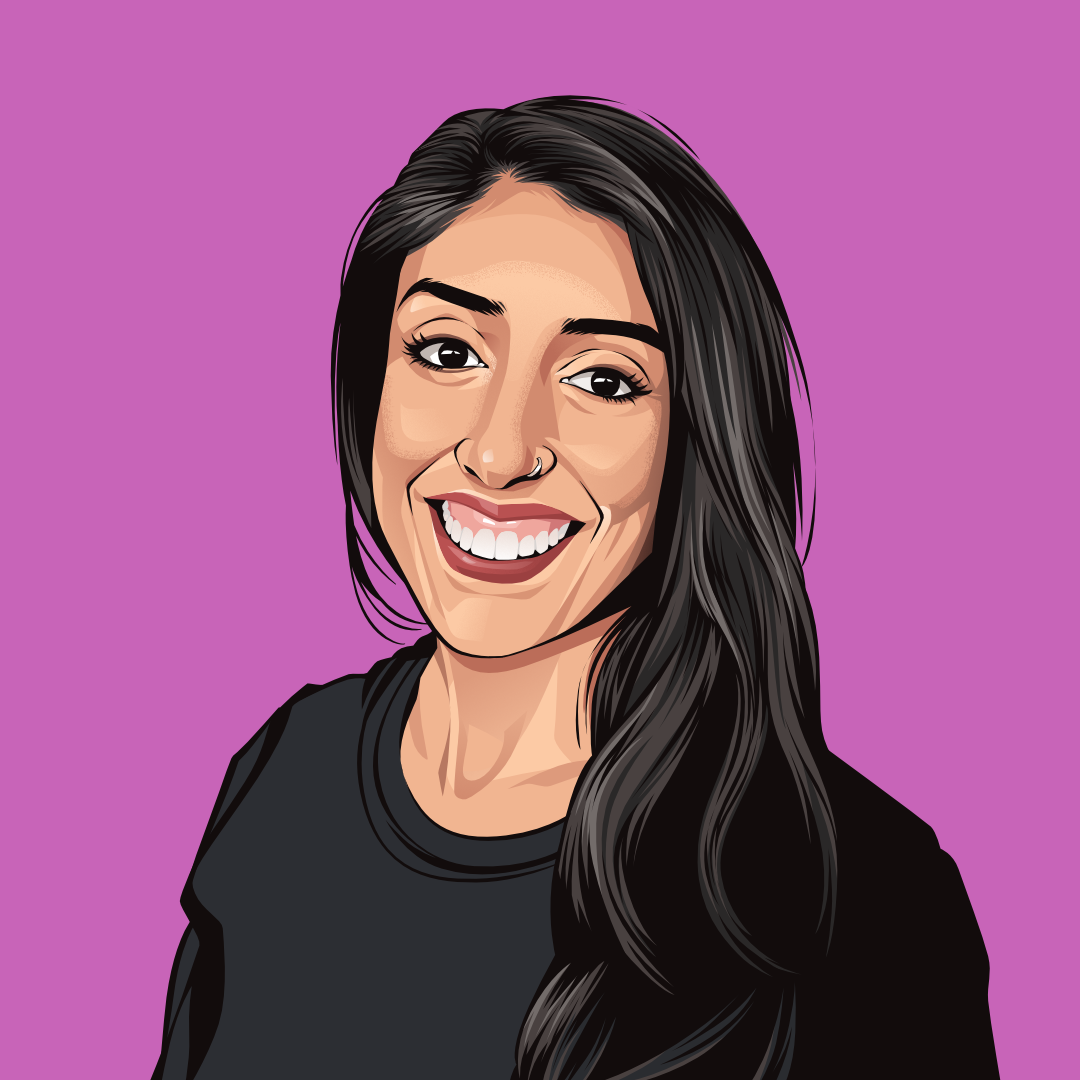
%20(9).png)
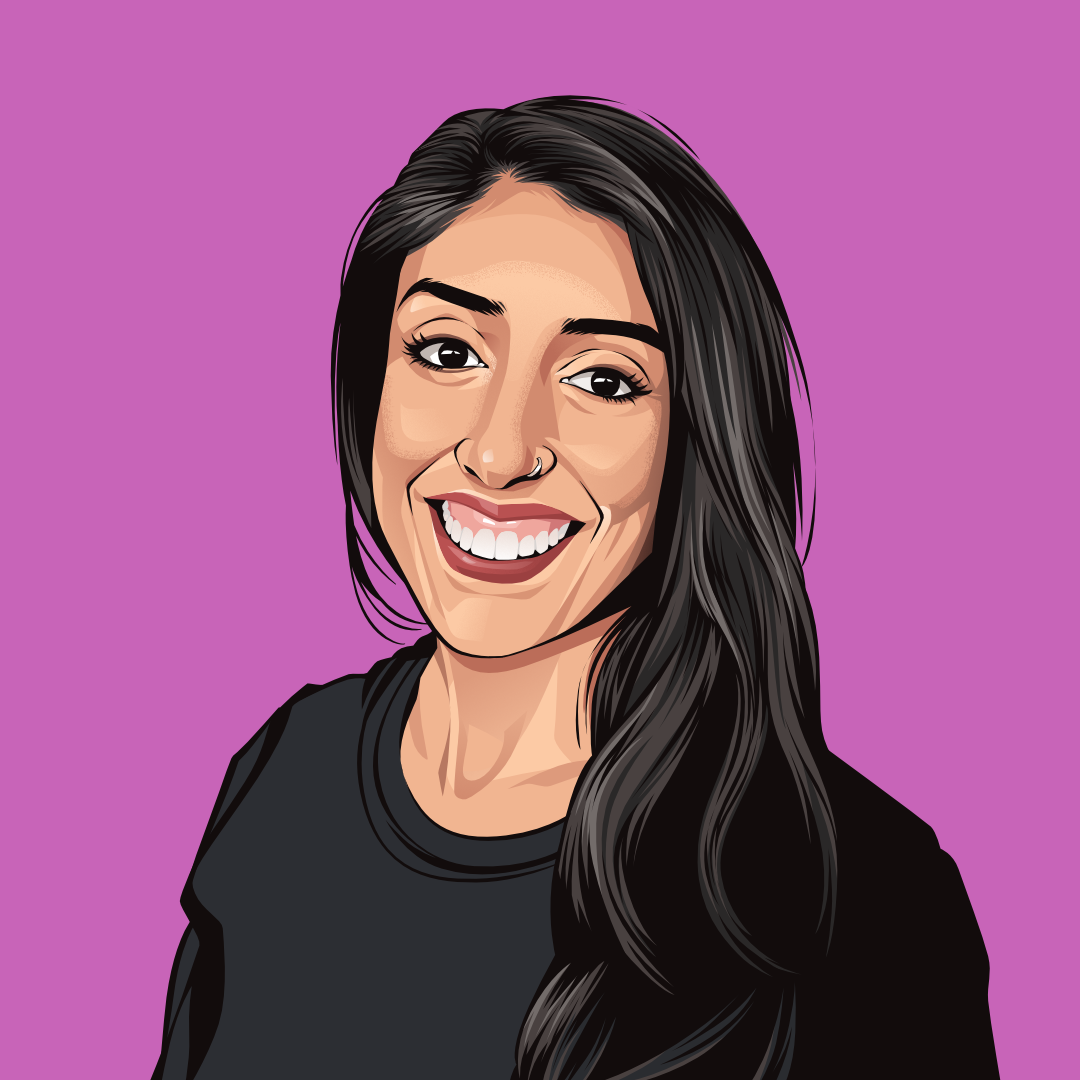
%20(8).png)
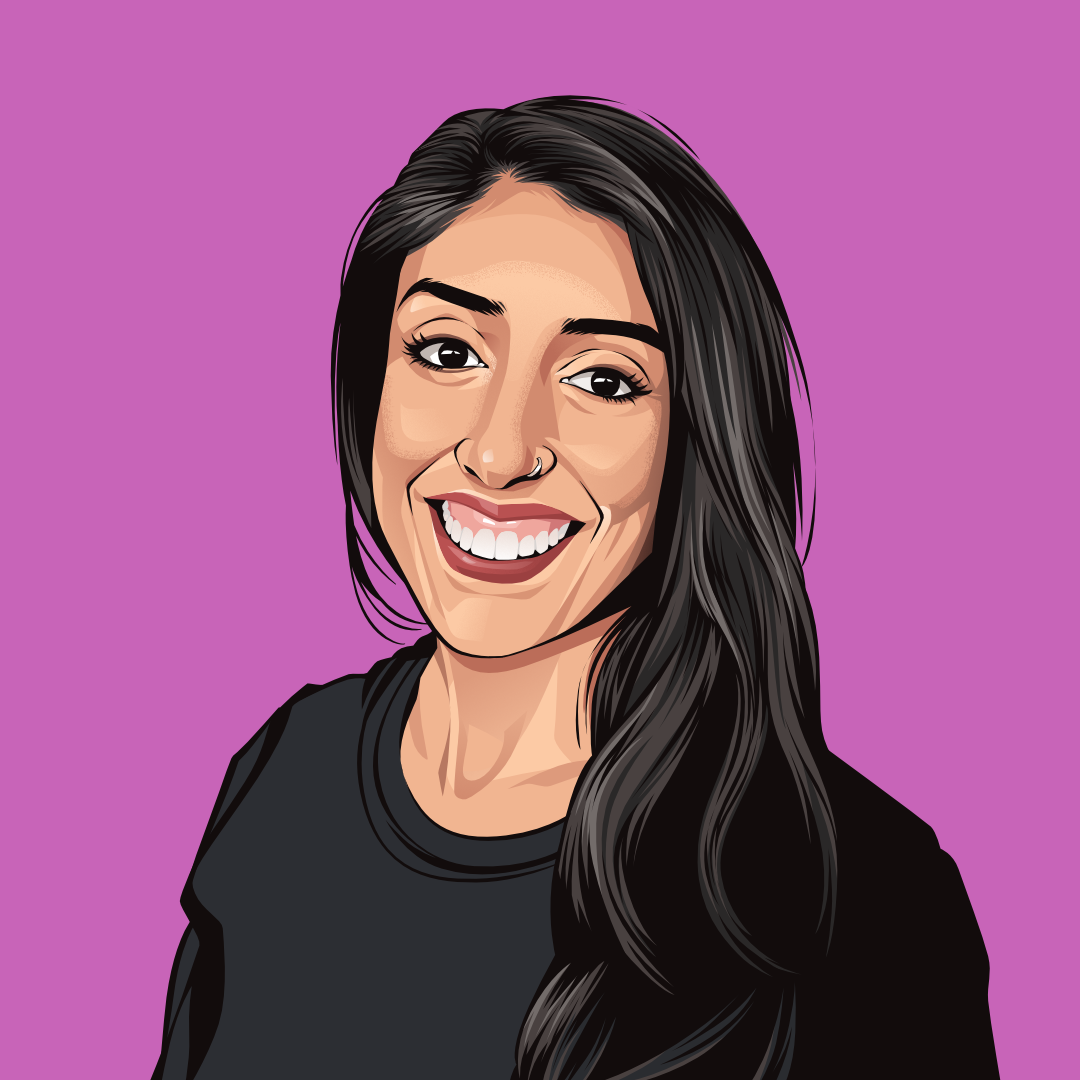